Structuring Tech Teams to deliver ambitious GenAI features
80% of organizations aren’t seeing any tangible enterprise-level impact from their use of Gen A .
At Inato, that’s a pitfall we want to avoid and we believe organizational structure is key to doing so. Our mission is to accelerate drug development by helping pharmaceutical companies and health organizations streamline their clinical trials. As we navigate an industry held back by manual processes and unstructured files, LLMs’ capabilities offer a game-changing opportunity to take a giant leap forward in our mission. Over the past two years, we have successfully transitioned from testing AI supplements to launching sophisticated AI-based products, such as our automated pre-screening tool (read more on that here).
With our growing experience in GenAI, we are now convinced that there are still many untapped opportunities to develop ambitious and impactful products. To fully unlock these exciting new capabilities and overcome their associated challenges, we wanted to take a step back to reassess what an ideal organization looks like for us.
To explore this problematic, I had the pleasure to interview several AI and data leaders from the Modern Data Network:
- Noé Achache (Head of AI Engineering at Theodo)
- Anas El Khaloui (Head of Data at HiPay)
- Emilie Gerardin (AI Lead at DisneyLand Paris)
- Sami Kaddani (ML Engineer at Too Good to Go)
- Kasra Mansouri (Head of Data at FinFrog)
- Thomas Moulin (Lead Data Scientist at Welcome to the Jungle)
Despite different contexts and industries, we all wrestle with the same fundamental questions:
- What are the specific challenges associated with GenAI?
- Where to draw the line between generalist software engineers and AI specialists?
- And ultimately, what is the optimal team organization?
Cela pourrait vous interresser
Partageons ensemble notre savoir
Nos prochains événements
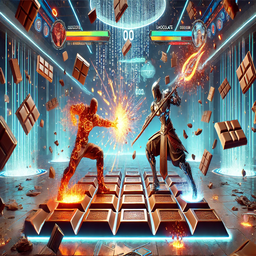
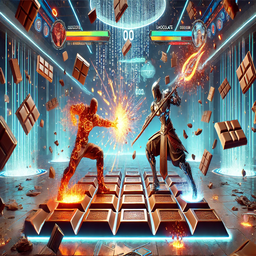
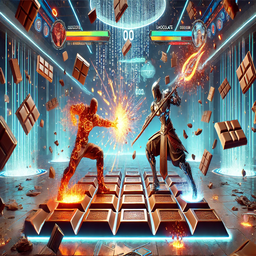